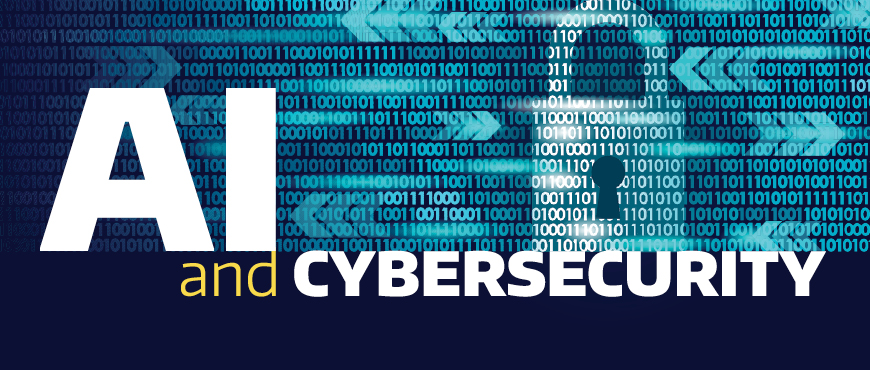
Our work in artificial intelligence (AI) and cybersecurity includes examinations of adversarial manipulation and the legal, policy, and other hurdles of cybersecurity research in AI.
Our work in artificial intelligence (AI) and cybersecurity includes examinations of adversarial manipulation and the legal, policy, and other hurdles of cybersecurity research in AI.
In this paper, presented at the 2018 Conference on Computer Vision and Pattern Recognition (CVPR 2018), researchers show that malicious alterations to real world objects could cause an object classifier to misread an image.
Research PaperPresented at the 12th USENIX Workshop on Offensive Technologies (WOOT '18), this paper explores physical adversarial attacks for object detection models, a broader class of deep learning algorithms widely used to detect and label multiple objects within a scene.
Research PaperAlthough deep neural networks (DNNs) perform well in a variety of applications, they are vulnerable to adversarial examples resulting from small-magnitude perturbations added to the input data. However, recent studies have demonstrated that such adversarial examples have limited effectiveness in the physical world due to changing physical conditions—they either completely fail to cause misclassification or only work in restricted cases where a relatively complex image is perturbed and printed on paper.
NewsPlease note: The Museum reopened on August 19th. Visitors must pre-book a free ticket in advance. Please visit https://www.sciencemuseum.org.uk/ for further details. Research exploring adversarial machine learning is on display at the Science Museum in London from June 2019 to October 2020 as part of “Driverless: Who is in Control?” This free exhibit includes a modified stop sign developed by a team of researchers to fool driverless cars into misidentifying it and asks “can self-driving cars see the world as well as you can?”
Event